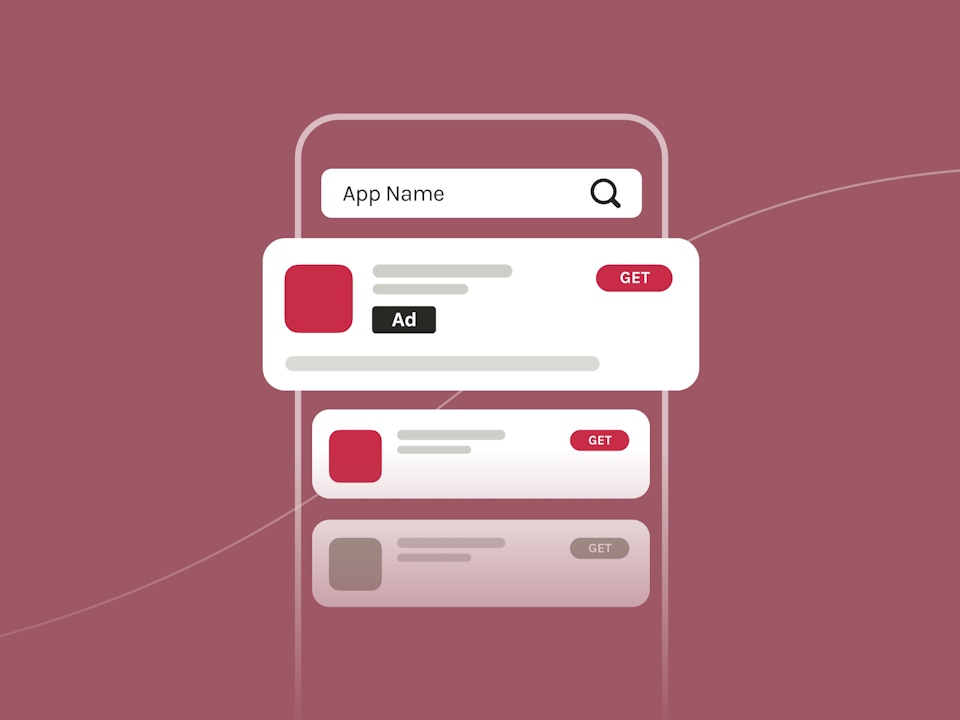
Measuring Cannibalization in Apple Search Ads
In the wake of Apple’s App Tracking Transparency (ATT) framework, many app developers have shifted more advertising budgets to Apple Search Ads (ASA), given its exemption from ATT. This shift has raised concerns about potential CPI increases and the risk of cannibalization within ASA campaigns. Understanding how to measure and mitigate cannibalization is crucial for optimizing your ad spend and maximizing ROI. In this blog, we will explore effective strategies for measuring cannibalization in Apple Search Ads, helping you make data-driven decisions to enhance your campaign performance.
The basics of Apple Search Ads & brand defense
Apple Search Ads allow app developers to bid on keywords to secure a top spot above organic search results, aiming to boost app downloads.
Although the bidding algorithm considers metadata and performance, many developers bid on competitor brand terms, leading to a practice of brand defense, that is bidding on their own brand terms to prevent traffic loss.
However, this raises concerns about cannibalization, where impressions and installs could have occurred organically.
Explore the key factors that should guide your choice to run brand defense campaigns
Cannibalization: A game of conversion differential
Cannibalization occurs when you pay for traffic or installs that would have happened organically. Cannibalizing impressions is straightforward: it’s about bidding on keywords where you already rank first organically. Cannibalizing installs, however, is harder to measure since it depends on whether a competitor can convert the paid impression into an app download.
The key factor here is the conversion differential between your brand and your competitors.
To estimate it, consider the following:
- Brand power and customer loyalty: Strong brands with high customer loyalty are less vulnerable to losing customers to competitors. Use user research to gauge your brand power and understand how users perceive your brand. Brands that resonate deeply with customers are more likely to retain them.
- Competitor notoriety and product similarity: Competitors unknown to most customers pose less threat. However, perceived similarities between products can lead users to switch. Even if products differ, similar appearances create risks of opportunistic behaviors and reconsideration.
- True search intent: Analyze search queries for broad match brand defense campaigns. For example, “[brand name] free” may indicate a search for a cheaper alternative. Sometimes, highly renowned brands reach a genre-defining status, causing users to search for the brand name but intending to find a similar product. This happens with names like “scrabble” or “mario kart,” which had significant brand volume before their official apps existed.
Formulate accurate conversion rate estimate
Once you have considered the factors that may impact your app’s conversion compared to competitors, modeling an actual cost for cannibalization requires formulating conversion rate hypotheses. This is the “hit or miss” part of the entire model and given conversion rates are closely guarded data (Apple does not even share organic conversion rate data at the keyword level with developers), formulating accurate hypotheses is a tough challenge. As such, the best route is probably to formulate multiple scenarios powered with “proxy” data to ensure they are not completely unrealistic.
The main data points to use in such a case are probably the keyword-level conversion rates you may obtain from your own Search Ads campaigns. When reasoning in abstract, it is only logical to imagine your own app’s conversion rate for your brand name is unlikely to be worse than any of your competitors bidding on your brand, and use it as the higher bracket competitors will have trouble reaching.
Inverting the logic and considering your app’s conversion rate for a competitor’s brand name is also a great way to determine an anchor for your hypotheses as to how this competitor might convert for your brand name.
Once you have determined your first anchors, remember to:
- Calculate a similarity score and refine your conversion differential hypotheses based on your own user research and the multiple data points that third party tools can offer.
- The app stores are all about comparing relevance. The multiple places where your app appears are as many opportunities to compare similarities and conversion performances with other apps.
Top organic search rankings, for instance, tend to reflect which apps among the most downloaded convert better than others for a specific keyword or query, and are a great hint to estimate conversion.
Model the cost of protection vs. cannibalization
Once you have set your competitors’ conversion rate estimates, modeling the actual cost of a defended install vs. the price of cannibalization becomes a simple question of maths. Assuming no impression is escaping the search ad inventory, the number of defended installs is the number of impressions you paid for that your competitors would have been able to convert into downloads; while cannibalized installs are the total number of installs you received from your Search Ads campaign minus the number of defended installs.
So, the cost per defended install becomes:
Cost Per Protected Install = Total Ad Spend / (Impressions • Competitor Conversion Rate)
Comparing this cost with the cost per install reported by Apple Search Ads can then lead you to estimate the protection premium you pay for a given campaign and what amount of your search ads spend actually goes to cannibalization. Unfortunately, knowing about these costs does not remove the dilemma of whether or not to bid on your top organic keywords, but it can at least help inform your decision to start, stop or change the budget you invest in specific Search Ads campaigns.
Use the cannibalization model efficiently & cautiously
While the question of measuring the exact cost of cannibalization has been central to Apple Search Ads since they were introduced, it is important to remember:
- Models such as the one presented here are meant to be a simplification of reality and help decision making, but need to be treated as such and nothing more. Don’t forget the model does not take into account certain parameters such as the percentage of users who will ignore the Search Ads placement by default, or the fact not all users end a search on the store by downloading an app.
- Also, the model’s accuracy will depend on the precision of your hypotheses, which means you should regularly test and retest Search Ads campaigns to maintain the data points behind your hypotheses up to date. Just as for organic performances, Apple Search Ads campaigns are conducted in an always evolving environment, and every keyword or creative change as well as many external factors can change an app’s conversion every day.
Want to know more about how AppTweak can help you calculate a similarity score between your app and your competitors? Start a free trial now!