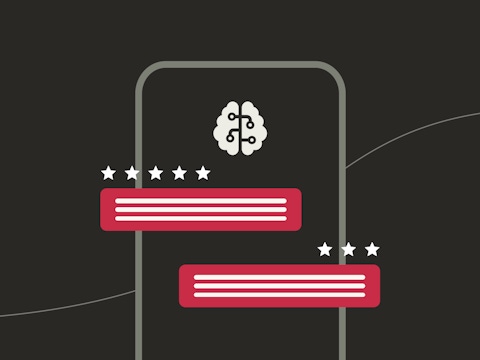
AI in ASO: Developing a New Model for Mining User Reviews
Since December 2022, ChatGPT has emerged as the flagship for artificial intelligence (AI) in the digital industry. The technology demonstrates impressive capabilities to produce qualitative human readable summaries out of a very large corpus of texts. In fact, not only can ChatGPT write concisely and clearly, it is also a great technology to reproduce a human answer in a very large variety of contexts.
With data science being at the heart of our platform, we, at AppTweak, took notice of the technology and started investigating how to leverage it. One crucial aspect of ASO where we thought of using ChatGPT is user reviews. Early experiments we’ve made for replying to reviews have convinced us to help our clients use the technology for that particular purpose.
With our own experience in building Atlas, the first store specific semantic engine, we saw an opportunity to put GPT to the test. We wanted to research if a dedicated AI model could be more performant to extract the most valuable insights left by users in app reviews on the app stores.
Identifying GPT technology limitations for a benchmark
When we asked ChatGPT what were the most common topics in the user reviews of Netflix for iOS, the first thing that struck us was that the answer was extremely intelligible and identified elements that seemed very likely to be mentioned by iPhone and iPad users.
But while ChatGPT is extremely skilled at writing summaries, and its speed opens new horizons for automation, the generic nature of the topic it highlighted also led to new questions:
- Could we trace back a topic to a specific corpus of reviews?
- Could we quantify the prevalence of a topic over another, both in general and when looking at a specific review?
- Could we retrace when a particular topic emerged in reviews?
As we investigated the answers, we found that ChatGPT, as a generalist technology, presents one particular flaw for the very specific topic we wanted to use it for. Its access to one of (if not) the largest pool of information in the world risked making it too smart for our purpose, as it might become tempted to produce answers based on information outside the App Store and Google Play. Specifically, it may get influenced by other sources, and could lead to an answer that contradicted factual reviews or did not reflect proportionally certain topics.
For ASO practitioners like us, this meant we could consider ChatGPT for suggesting which topics to look for in reviews, but using it for more directional insights would, at the very least, require a good amount of supervision and control on input.
Since this contradicted our initial ambition, we decided to try another model.
Find out how you can use ChatGPT to optimize your ASO efforts
Applying semantic machine learning to user reviews
With a very large collection of app reviews stored in our databases (we collect user reviews from all the apps and all countries followed by our clients), we were able to experiment with unsupervised machine learning techniques that would focus on word frequencies and their associated semantics.
With this method, not only was the input more specific but the only task for our operator would be to choose a number of distinctive topics for the model to identify. From there, the model is able to determine by itself what were the “x” most distinctive topics in the total amount of reviews given to it, and then look at any specific review and give it a score for each topic it identified.

This, in turn, gave us satisfaction with the limitations we had found with ChatGPT:
- Any topic can now be traced back to a subset of reviews read by the model.
- Any past and future review can also receive a score for each topic.
This implies that we can not only identify more than one topic per review but also monitor the quantitative evolution of a topic over time, allowing for alerts when a particular topic suddenly appears.
This last benefit is not to be underestimated, as it also helps overcome one of the most frequent biases in human review monitoring, which is to instinctively look for topics we expect to find.
When testing our model on Netflix’s 1-star and 2-star reviews in the US (iOS), we were surprised to find that the topic of removed content had become particularly prominent around September 2022 after Netflix removed the Vampire Diaries series from their platform.
Turning app review topics into marketing successes
Of course, knowing the most prominent topics is not enough to make an app successful. Nevertheless, it is an essential first step.
- Understand the pain points identified by users: Being aware of product issues is extremely beneficial for product managers who need to ensure the app’s quality (and deliver healthy product vitals). Putting a score on user suggestions can also help make data-based decisions when it comes to adjusting a product roadmap.
- Emphasize positive reviews: App store reviews and ratings have a much larger impact these days. Not only do Google and Apple often remind app developers that their average rating can be a crucial factor for them to be featured on the App Store or Play Store, but studies have also shown store users can be very mindful of user reviews before downloading an app.Apptentive’s 2020 report highlighted how a jump from a 3-star average rating to 4-star was likely to deliver a 92% increase in conversion rate. Highlighting positive reviews in app store screenshots has also become somewhat of a best practice for apps looking to inspire trust in their product or wanting to highlight a particular feature.
Our own experience has also led us to great success when a review mining analysis helped identify a comparative advantage for one of our managed services clients. We leveraged this advantage as inspiration for designing a custom product page on iOS, which we then used in Apple Search Ads campaigns to target competitors’ brand names. This resulted in a 58% conversion rate improvement and lowered the Cost Per Install by nearly 40%.
Our team has since continued experimenting with our semantic review mining model, making improvements to assist apps with a limited number of reviews per month in a given market. We also made a few thought experiments on how to compare review topics across app clusters. All in all, this has comforted our belief in the potential of AI for ASO, and will continue adding more data-science powered functionalities in our platform.
If you want to keep learning about how we use AI at AppTweak and benefit from our latest functionalities, sign-up for a 7-day free trial.