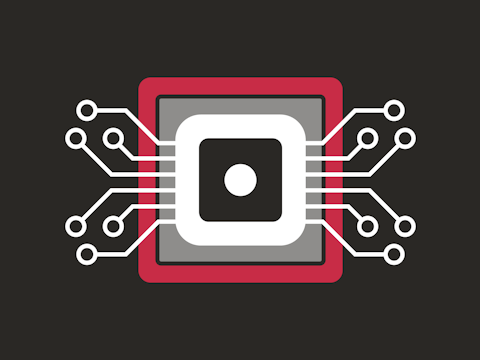
Learnings Gained from Reverse Engineering the App Stores
Marketing on the app stores is all about finding a match between the right customer and the right app. Unfortunately, the quest for user acquisition is not a smooth path, mainly because of one specific hurdle – the black box algorithm run by Apple and Google.
The marketplaces are the middlemen in the acquisition funnel. They create the rules, but we don’t perceive exactly how they work. In this blog, we’ll present the learnings drawn by reverse engineering the app stores. We shall further elaborate on how you can benefit from our learnings via the AppTweak tool.
How do the stores process app metadata and user behavior?
Translate the app stores into data points
The main challenge here is to understand how the stores convert and match an app’s metadata to a user’s behavior. We already know the search results from keyword queries and also which metadata are available. So now we wanted to find out how this matches user behavior and, as a result, decipher the logic behind an algorithm on the app stores.
The first point is to look at the data and understand which pairs of applications and keywords are valuable in the eyes of the stores.

Our approach was to mimic the algorithm itself. So we tried to reproduce the results, as Apple and Google are doing with our data points, and then analyze our algorithm. We ended up positioning all the keywords and apps in one single map per store, whereon the exact positions of each app and keyword are computed by a machine-learning algorithm trained on billions of data points.
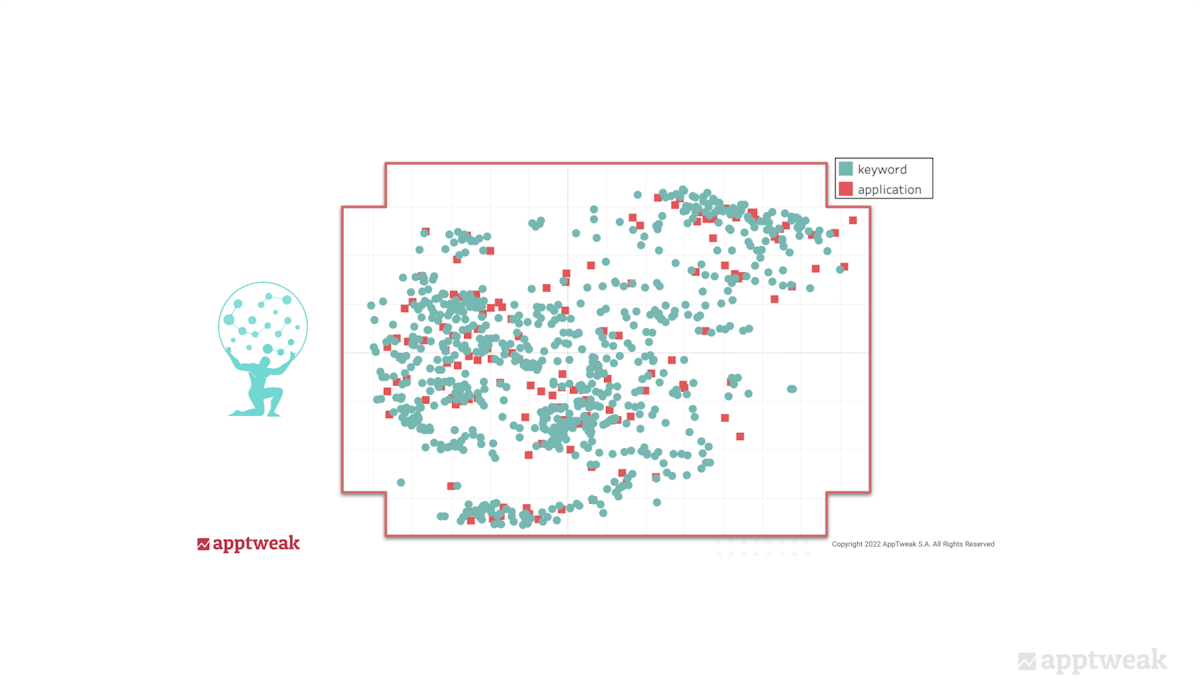
Release of Atlas – first App Store semantic engine
From this algorithmic representation, Atlas was born – the first App Store semantic engine that helped us understand how the app stores group similar apps together, which keywords the app stores consider relevant to certain apps, and much more.
Simply said, Atlas is a mapping of all apps and keywords according to their store semantic meaning. This foundational model ensures that:
- Similar keywords are positioned close to each other
- Similar apps are positioned close to each other
- The position of apps and keywords are comparable
The distance between an app and a keyword on this representation is a measurement of the similarity between these two.
Identify app clusters and make sense of them
By mapping apps via Atlas, we get something similar from the categories of the App Store, even though Atlas never heard about the notion of categories before.
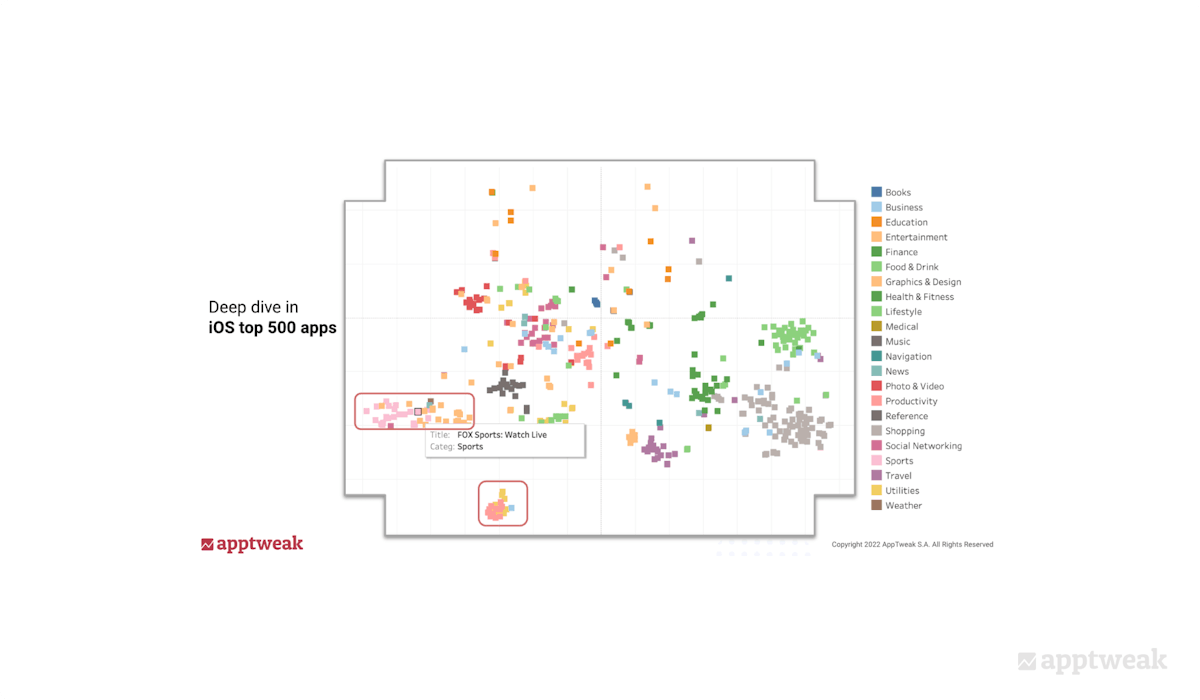
Looking now at the very bottom of the map, we can see a clear cluster that is strictly made of VPN apps, but there is no VPN category on the app stores. The VPN apps are either classified in the Utilities or Productivity category. Atlas allows us to have more granular categories (which we call AppDNA/GameDNA) than the app store categories. Furthermore, the overlapping of the Entertainment and Sports categories that Atlas created is made of Sports Broadcasting apps!
As a marketer, you can now identify which apps are close to yours. Since our findings were translated from users’ intentions, it means that you can now understand app similarities from a user’s point of view. AppTweak released new features from these more granular categories – the top chart and competitors selection per AppDNA or GameDNA.
Understand keywords as an algorithm does
In the eyes of app stores’ algorithms, keywords are much more than a sequence of characters. The map hereunder illustrates it nicely – taking the example of the words lemon, lime, and scooter. From a language perspective, lime and lemon are very similar. But from the store algorithm, it is lime and scooter that are the most identical.

This demonstrates how we understand the app stores’ semantics by reverse engineering their algorithm. Moreover, this confirms that natural language and store language are different. It also highlights how the stores are able to identify acronyms or typos.
Therefore, you shouldn’t only look at keyword combinations that you immediately identify when doing keyword optimization either for ASO or Apple Search Ads (ASA). As a matter of fact, store algorithms are trained to see keywords as more than a simple string of characters but objects that carry meanings and intents, which are conveyed by user behaviors.
Decrypt keyword relevancy & leverage its use in ASO & ASA
A key insight from our state-of-the-art mapping lies in the distance between a keyword and an app. The distance expresses a measure of correspondence between a keyword and an app. Keeping that in mind, we developed a new metric called Relevancy Score to help you ensure that keywords in your app metadata actually target the right users who will find value in your app. This metric will help you avoid keywords that refer to different types of apps that provide contrasting features or functionalities to your own.
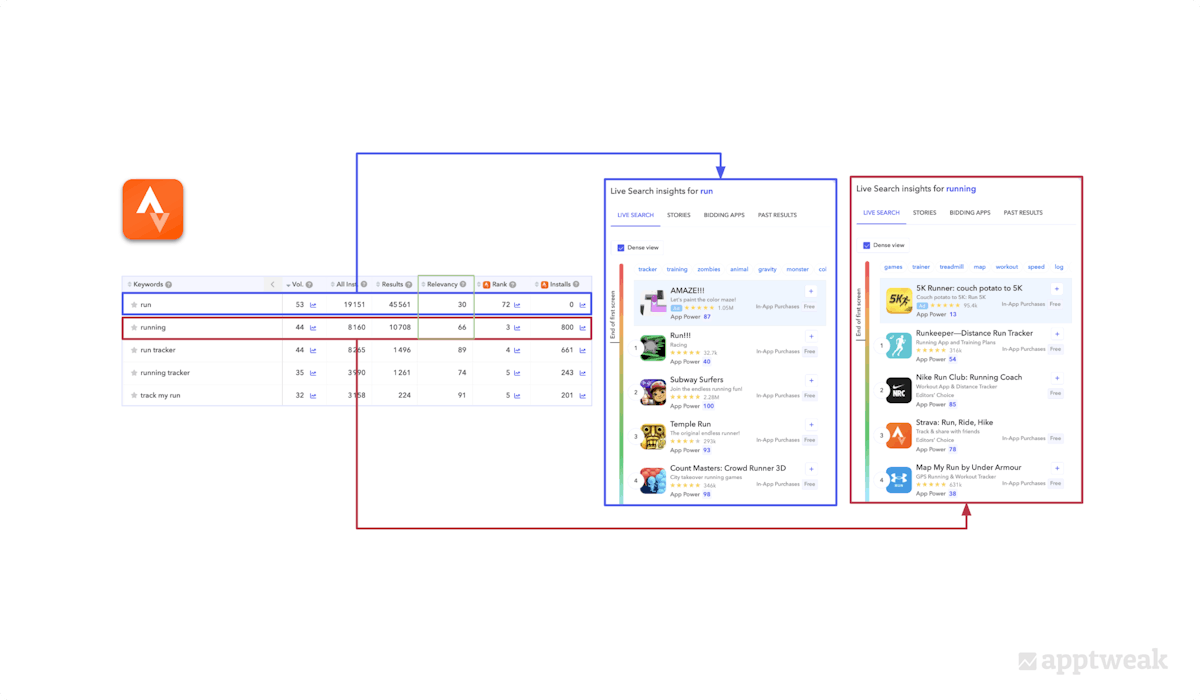
With our Relevancy Score, you can:
- Identify opportunities and/or prioritize which terms to test for ASO or Apple Search Ads (ASA)
- Set up sanity checks and identify counter-intuitive terms to add as negative keywords in your Apple Search Ads campaigns
- Review the relevancy of other apps for your top organic keywords to evaluate the threat level they represent and start modeling a defense strategy with ASO and ASA
TLDR
On the app stores, the algorithms that match a user’s intent to an app metadata are a black box. In the quest of understanding the dynamics of the stores, we mimicked the algorithms and acquired some key learnings:
- The natural language is different from the stores’ semantics. In the eyes of the app stores, keywords are more than a simple string of characters but objects that carry meanings and intents conveyed by user behaviors.
- Thanks to Atlas, we developed a unique metric called Relevancy Score to measure the correspondence between a keyword and an app, that is how relevant a keyword is to your app.
- The creation of AppDNA and GameDNA taxonomy, a more precise categorization than the one provided by the stores. Leveraging AppDNA, you can also visualize the top charts and competitors selection per DNA to have a better view of your competitors!
Discover how AppTweak can help you boost your app downloads with actionable insights in a simple interface.